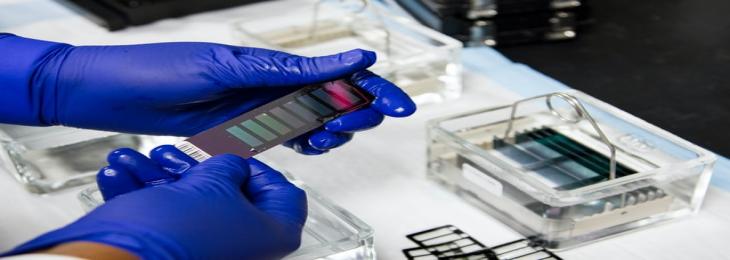
This suggests that patients might benefit from customized treatments with shorter turnaround periods and reduced costs.
The status of molecular pathways involved in the development of colorectal cancer, as well as important cancer mutations, must be established to treat the disease swiftly and efficiently. Current approaches entail pricey genetic testing, which may be time-consuming. Furthermore, researchers from the University of Warwick's Department of Computer Science have been investigating how deep learning might be used to forecast the state of three major colorectal cancer molecular routes and hyper-mutated tumors. A significant aspect of the approach is that no manual comments on digital pictures of cancer tissue slides are required.
The researchers provide a different iterative draw-and-rank sampling technique that can choose representative sub-images or tiles from a whole-slide picture without the requirement for a pathologist to provide precise comments at the cellular or regional scale. The developed model, in essence, can use the potential of raw pixel data to identify clinically significant mutations and pathways for colon cancer without any need for human intervention.
Iterative draw-and-rank sampling uses a deep convolutional neural network to find picture areas that are most prognostic of important molecular markers in colorectal tumors. Iterative draw-and-rank sampling allows for a systematic and data-driven study of the composition of the cells of picture tiles, which is highly predictive of colorectal molecular mechanisms. The efficiency of iterative draw-and-rank sampling was also tested by the researchers, who discovered that their algorithm was much more accurate than recently published techniques for predicting the three primary colorectal cancer molecular pathways and important mutations. This indicates that the novel algorithm might be utilized to stratify patients for targeted treatments at lower costs and faster turnaround rates than sequencing or special stain-based methods following large-scale validation.
These results indicate that iterative draw-and-rank sampling might be used to identify patients who are likely to benefit from targeted treatments, at a cheaper cost and with a shorter turnaround time than sequencing other special marker-based methods. The researchers will now perform a major multi-centric validation of this method in order to open the route for its clinical use.