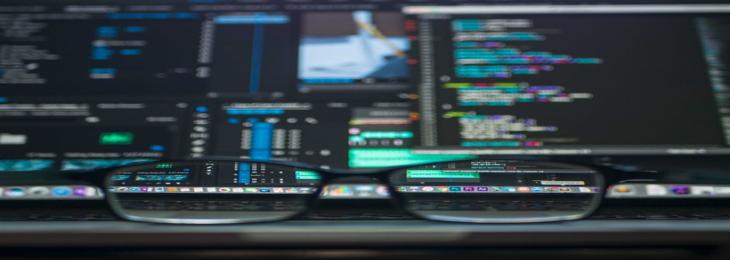
Deep learning is gaining popularity in underwater acoustics for upgrading sonar systems to detect submarines and boats in danger or prohibited waters. Furthermore, noise interference from the dynamic marine ecosystem makes detecting targeted ship-radiated sounds difficult.
Deep learning is a machine-learning technology that recognizes patterns by using artificial neural networks brain-inspired. Depending on the information stored in the previous layer, each layer of artificial neurons, or nodes, learns a unique collection of attributes. ABNN employs an attention module to replicate cognitive process features that allow humans to concentrate on the most significant bits of an image, language, or another pattern while tuning out the rest. This is performed by giving more weight to specific nodes in the machine-learning process in order to improve specific pattern aspects.
The researchers tested two ships in a shallow, around 135-square-mile area of the South China Sea, using an ABNN system in sonar technology for targeted ship detection. They evaluated their findings to those of a standard deep neural network (DNN). More than 17 interfering vessels in the experimental area were identified using radar and other devices. They discovered that the ABNN improves its predictions significantly as it gravitates toward traits that are closely connected to the training goals. The network's detection grows more obvious as it cycles through the whole training dataset, emphasizing the weighted nodes and ignoring irrelevant information.
While the ABNN accuracy of recognizing ships A and B individually was somewhat higher than the DNN (98 % and 97.4 %, respectively), the ABNN accuracy of recognizing both ships in the same area was much higher (74 % and 58.4 %). A classic ABNN model is typically trained using multiship data for multiple-target recognition, but this can be a complex and computationally expensive procedure. The team trained the ABNN model to recognize each target individually. As the network's output layer is enlarged, the individual-target datasets combine.