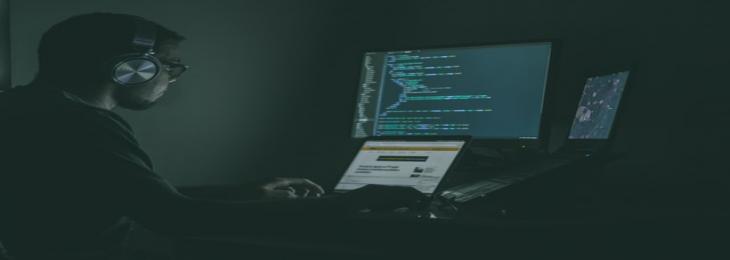
Researchers developed a modern machine learning-based framework to enrich the security of computer networks inside vehicles without deflation of performance.
In collaboration with a team of international experts from Virginia Tech, the University of Queensland, and the Gwangju Institute of Science and Technology, researchers in the U.S. optimized a renowned cybersecurity strategy known as the moving target defense.
Usually, in-network attacks, opponents take their time to look at everything and choose their motives. To counter this, the defense team needs to change the IP addresses fast enough, the information allocated to the IP is lost immediately, and then must be retrieved for backup. DESOLATOR, which represents the distribution of in-depth resources based on the learning and delivery framework of targeted deployments, helps the automotive network to identify the absolute frequency of mixing IP and bandwidth allocation to deliver effective, long-lasting protection. Previous studies keep the uncertainty high enough to prevent potential attackers unless it is too expensive to keep, while the final detection prevents a downfall in critical network areas. Existing legacy in-vehicle networks work very well, but they were not designed for security.
The current technology facilitates lightweight protection with fewer resources are used for increased protection. The use of fewer resources to protect automotive systems and devices connected to vehicles while maintaining the same quality of service is an additional benefit. The team of researchers from the University of Queensland and the Gwangju Institute of Science and Technology used intensive learning to gradually streamline the performance of the algorithm depending on functions, such as the number of discarded packets and exposure time to ensure that DESOLATOR takes safety and efficiency into critical thinking.
In addition, DESOLATOR is not limited to shuffling frequency and bandwidth distribution. Since this approach exists as a framework based on machine learning, some researchers can modify the process by following various objectives within the problem area.