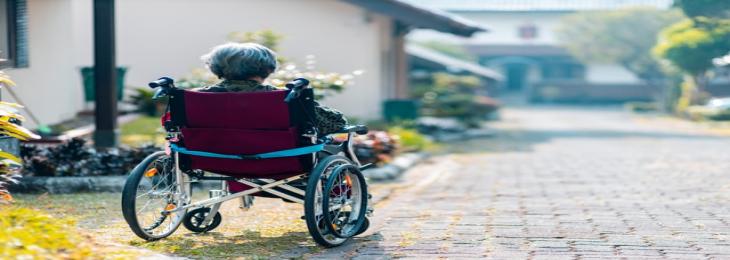
The deep learning-based model used a modified version of the popular fine-tuned ResNet 18 - residual neural network to categorize functional MRI images extracted from 138 participants.
Alzheimer's disease is one of the most common cause of dementia, resulting for around 70% of cases, according to the World Health Organization. Around 24 million people are affected globally, with this number expected to double every two decades. Researchers from Lithuania's Kaunas university created a deep learning-based system that can predict the development of Alzheimer's disease from brain scans with an accuracy of around 99%. The methodology was designed by evaluating functional MRI scans from 138 participants and excelled in previously developed methods in terms of accuracy, specificity, and sensitivity.
Mild cognitive impairment (MCI) is the stage of mental impairment that occurs between normal ageing and dementia, is one of the possible early indicators of Alzheimer's disease. According to a project researcher, functional magnetic resonance imaging (fMRI) can be utilized to recognize brain regions related to the start of Alzheimer's disease based on previous studies. Although theoretically possible, manually processing fMRI scans in order to identify the effects associated with Alzheimer's disease not only takes time but also requires detailed knowledge. The use of Deep Learning and other AI technologies can significantly accelerate this process.
A deep learning-based model used a version of the ResNet 18 (residual neural network) to identify functional MRI scans collected from 138 participants. Images were classified into six groups, varying from healthy to mild cognitive impairment (MCI) to Alzheimer's disease. The model efficiently found the MCI characteristics in the given dataset, obtaining the best classification precision of 99.99 % for early MCI vs. AD and 99.95 % for late MCI vs. AD.
According to the lead researcher, the above-described model may be combined into a more complicated system, assessing numerous different factors, such as monitoring eye-movement tracking, facial reading, speech analyzing, and so on. Such technology may subsequently be used for self-checking and notifying people to pursue expert help if that is causing them worry.