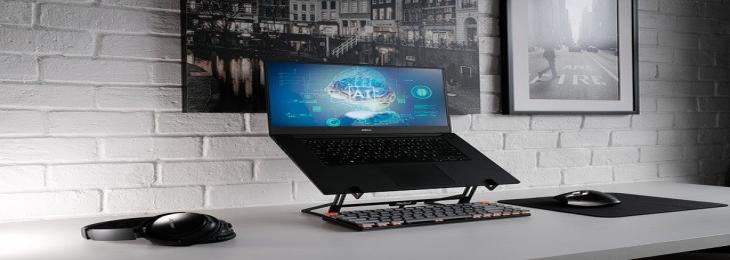
Researchers from Cornell University applied machine learning to classify organisms based on their functions and then used this data to forecast how genes are transmitted in them with relatively close accuracy, a strategy that might be used to halt the development of antibiotic resistance.
Genes are not just passed down through families. Bacteria can send genes to one another or pull them up from their environment by a process known as horizontal gene transfer, which is a major contributor to the emergence of antibiotic resistance. Many unique features are shared as a result of gene transfer. Furthermore, scientists have yet to discover why certain bacteria participate in gene transfer and others do not.
Rather than validating particular theories, the team examined bacteria genomes and their many functions which can vary from replication of DNA to metabolism of carbohydrates to uncover signals that indicate who was exchanging genes and what was fueling these exchange networks. The team deployed a variety of machine-learning models, which each extracted multiple variables from the information. They were able to determine numerous networks of distinct antibiotic resistance genes among strains of the same organism as a result of this. The researchers concentrated on organisms linked with soil, oceans, and plants for the research, but the algorithm is also well-suited to study human-related pathogens and organisms such as E. coli, Acinetobacter baumannii, as well as inside localized ecosystems such as the gut microbiome.
They discovered that when applied to antibiotic resistance genes, machine-learning algorithms were highly effective. One of the most startling discoveries was that the algorithm projected many previously unobserved antibiotic resistance exchanges between human-related bacteria or viruses. These possibly unnoticed transfer events were almost entirely limited to human-associated bacteria in the oral microbiome or gut microbiome.